📺 Fabian Coscia seeks to shed new light on cancer cells
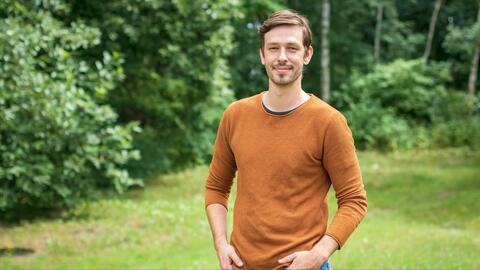
For most cancers, there are therapies that work well for many patients. But for some, that’s not the case. This is particularly true with recurrent cancer where the tumors are resistant to the usual chemotherapeutic agents. In these instances, it can help to take a closer look at the cancer cells – not just studying the genes, but also investigating their end products, the proteins. “Proteins are the actual functional carriers in the cells,” explains Coscia. “They determine whether a cell becomes diseased or not.”
So far, oncology has looked almost exclusively at a tumor’s genetic profile in the search for targeted drugs. “But the information about mutations in certain oncogenes doesn’t tell us anything about what is behind the mutation,” says Coscia. “Namely, whether and to what extent the cancer cell actually produces the corresponding mutated protein and what influence this has on the signaling pathways in the cell.” He therefore believes that the key to treating tumors that have previously proven resistant to therapy lies in a combination of genomics and proteomics.
“Just the environment I was looking for”
“Translational proteomics research relies on technological advances and medical collaborations,” says Coscia. “So a technology-focused institute like the MDC, where you always have access to the latest innovations and are surrounded by a fantastic medical center is just the environment I was looking for.” Coscia is now setting up his own lab for the first time and is very much looking forward to working with his colleagues at the MDC.
Coscia studied molecular biotechnology at the Technical University of Munich (TUM) and developed an early interest in translational research. “In addition to conducting basic research on cell cultures, I was soon working with patient biopsies,” says the 34-year-old. He earned his PhD at the Max Planck Institute of Biochemistry (MPIB) in Martinsried with a focus on the proteome of ovarian carcinomas, for which he was recognized with the Walter Schulz Stiftung’s Cancer Research Award in 2019. Coscia then moved to the University of Copenhagen as a postdoc in Matthias Mann’s lab. There, over the past four years, he and his colleague Andreas Mund have developed a method called Deep Visual Proteomics, which involves using high-resolution imaging to analyze the protein patterns of cancer cells. “The ‘deep’ comes from deep learning, which is a form of artificial intelligence, and ‘visual’ because of the microscopy techniques,” explains Coscia.
Well-trained AI sees more than the human eye
We use AI to identify cells and tissue regions of morphological interest under the microscope and to cut them out in an automated way.
AI can often “see” more than even the most skilled researcher. This is because well-trained AI is far superior to the human brain when it comes to comparing tens to hundreds of millions of images. “We use AI to identify cells and tissue regions of morphological interest under the microscope and to cut them out in an automated way,” explains Coscia. The resulting biopsy contains fewer than 100 cells, the proteins of which are then analyzed. “We can’t yet measure the proteome of single cells in tissue samples,” says Coscia. “But that’s what we ultimately want to achieve.”
In the BMBF-funded project MSTARS (Multimodal Clinical Mass Spectrometry to Target Treatment Resistance), Coscia and his team at the MDC now want to apply and further develop the Deep Visual Proteomics technique with samples taken from solid tumors. MSTARS is a research consortium comprising the MDC, Charité – Universitätsmedizin Berlin, the Max Planck Institute for Molecular Genetics (MPIMG) and Humboldt-Universität zu Berlin.
Using protein profiles to track disease progression
One of the attractions of the new method is that protein patterns from biopsy samples can be analyzed retrospectively, because – once embedded in paraffin – the proteins are kept stable for a very long time. “This allows us virtually turn back time and take the patients’ samples out of the archive again after 10 to 20 years, analyze them, and thus follow the entire course of the disease via the protein profile, looking for correlations with clinical progression data,” explains Coscia. And this is still possible even if just a few cancer cells are present in a sample.
Text: Catarina Pietschmann
Further information