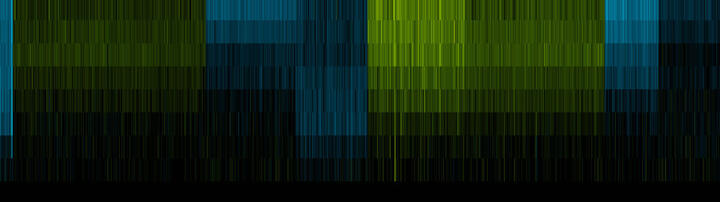
Landthaler Lab
RNA Biology and Posttranscriptional Regulation
Profile
Post-transcriptional regulation is highly versatile and adaptable in exploiting cellular time and space. RNA-binding proteins play a key role in the regulation of spatial and temporal changes in protein synthesis through control of transport, translation and decay of mRNA. Deregulation and failed coordination of these mechanisms contribute to the pathophysiological development and conditions.
Transcriptome-wide high-resolution maps of RNA-protein contacts allow us to study how these interactions control mRNA fate [Hafner & Landthaler et al., Cell 2010; Rybak-Wolf et al., Cell 2014]. Different next generation sequencing approaches are being applied to examine the function of RNA-binding proteins in mRNA biogenesis, translation and decay [Murakawa et al. Nature Communications 2015].
In addition, we have developed a UV crosslinking and oligo(dT) purification approach to identify the mRNA-bound proteome using quantitative proteomics. We use this method to monitor dynamic changes of the mRNA-protein interactome to capture differentially binding proteins as a consequence of intra- and extra-cellular signals. [Milek et al., Genome Research 2017].
Team
Research
Our main interest is the understanding of post-transcriptional regulatory networks that control gene expression. Post-transcriptional regulation is highly versatile and adaptable in exploiting cellular time and space. microRNAs and RNA-binding proteins play a key role in the regulation of spatial and temporal changes in protein synthesis through control of mRNA transport, storage and translation. Deregulation and failed coordination of these mechanisms contribute to the pathophysiological development and conditions. A prerequisite for a systems level understanding of post-transcriptional regulation is a transcriptome-wide high-resolution map of RNA-protein contacts that allows us to study how these interactions control the fate of mRNAs.
We are using a novel crosslinking-immunoprecipitation approach (PAR-CLIP) in combination with “deep-sequencing” to identify functional RNA-protein interactions at a nucleotide resolution (Hafner & Landthaler et al. [2010] Cell, 141). By using these RNA-protein interaction maps and combining them with cell-based and biochemical assays, we are aiming to understand the coordinated and combinatorial assembly of microRNAs, RNA-binding proteins and helicases on their target mRNAs as well as the structures and mechanisms guiding mRNA maturation, localization, turnover and protein synthesis in response to stress and environmental signals.
Publications
News
SARS-CoV-2 gene expression studies
Single-cell RNA-sequencing of lung and blood from SARS-CoV-2 infected Syrian hamsters: Temporal omics analysis in Syrian hamsters unravel cellular effector responses to moderate COVID-19
Seurat/R objects and tables for the data analysis described on github (https://github.com/Berlin-Hamster-Single-Cell-Consortium/Single-cell-sequencing-of-COVID-19-pathogenesis-in-golden-Hamsters-)
Additional files are provided in the download folder here: https://filetransfer.mdc-berlin.de/?u=kDhdyhMM&p=6FzDwbfX
SARS-CoV and SARS-CoV-2 infections of human cell lines: Transcriptomic profiling of SARS-CoV-2 infected human cell lines identifies HSP90 as target for COVID-19 therapy
GEO entry
Raw sequencing data, as well as readcount tables from the bulk and single-cell sequencing experiments can be downloaded from the GEO entry: https://www.ncbi.nlm.nih.gov/geo/query/acc.cgi?acc=GSE148729
Online browsing of Calu-3 single-cell RNA-seq data
The Calu-3 scRNA-seq data can be interactively browsed here: https://shiny.mdc-berlin.de/COV/
Count tables scRNA-seq
For scRNA data, count tables for cells with more than 1000 detected genes are provided. Within the manuscripts, only cells with more than 2000 detected genes have been analyzed. The following R code can be used to convert the count tables to a Seurat object:
library(Seurat) raw_counts <- read.table(file="GSE148729_Calu3_scRNAseq_morethan1000genes_rawcounts_tr.txt",sep="\t“) meta_data <- read.table(file="GSE148729_Calu3_scRNAseq_morethan1000genes_metadata.txt",sep="\t",header=TRUE,dec=".",row.names="cell_id“) seu <- CreateSeuratObject(counts =t(raw_counts), meta.data = meta_data)
Supplementary data and Seurat/R objects
Additional files are provided in the download folder here: https://filetransfer.mdc-berlin.de/?u=CVXckugR&p=MACT6Xw9
Supplementary tables
Data set overview: SupplementaryTable1.xlsx
Percentage of viral reads: SupplementaryTable2.xlsx
Viral transcripts reads: SupplementaryTable3.xlsx
Statistics scRNA-seq: SupplementaryTable4.xlsx
Oligos: SupplementaryTable5.xlsx
RNA-seq gene normalized expression values (fpkm)
SupplementaryData1_Caco2_polyA_fpkm.tsv
SupplementaryData2_Calu3_polyA_series1_fpkm.tsv
SupplementaryData3_Calu3_polyA_series2_fpkm.tsv
SupplementaryData4_Calu3_total_RNA_fpkm.tsv
SupplementaryData5_H1299_polyA_fpkm.tsv
Seurat objects scRNA-seq
200406.Seurat_H1299_CoV_1000_Merged.rds
200408.Seurat_Calu_CoV_1000_Merged.rds
Diffusion embedding, intron and RNA velocity data
This is the embedding used in figure 5, and the corresponding mature mRNA / intron / RNA velocity data. A meta data file with an embedding calculated without viral genes is also provided (not displayed in the manuscript)
diffusion_embedding_meta_data.txt.zip
diffusion_embedding_withoutviralgenes_meta_data.txt.zip
intron_matrix.rds
mrna_matrix.rds
velo_matrix.rds
Viral sequences and annotation used for scRNA-seq analysis
Accession numbers: AY310120 for SARS-CoV, MN908947 for SARS-CoV-2
viral_sequences_annotations.zip
Alignements of small RNA-seq data to the viral genomes
Calu3_smallRNAseq_SARS_alignements.zip
Differential expression lists
Differential expression calculation outputs from edgeR, with log(2) fold changes, p-value, logCPM and FDR, of some comparisons infected/mock from the polyA datasets
Series2, Calu-3 cells, 12hpi SARS-CoV vs. 12h mock: DE_Calu3ser2_S1.12h_mock.12h.txt.zip
Series2, Calu-3 cells, 12hpi SARS-CoV-2 vs. 12h mock: DE_Calu3ser2_S2.12h_mock.12h.txt.zip
Series1, Calu-3 cells, 24hpi SARS-CoV vs. 24h mock: DE_Calu3_S1.24h_mock.24h.txt
Series1, Calu-3 cells, 24hpi SARS-CoV-2 vs. 24h mock: DE_Calu3_S2.24h_mock.24h.txt
Series1, Caco-2 cells, 24hpi SARS-CoV vs. 24h mock: DE_Caco2_S1.24h_mock.24h.txt
Series1, Caco-2 cells, 24hpi SARS-CoV-2 vs. 24h mock: DE_Caco2_S2.24h_mock.24h.txt